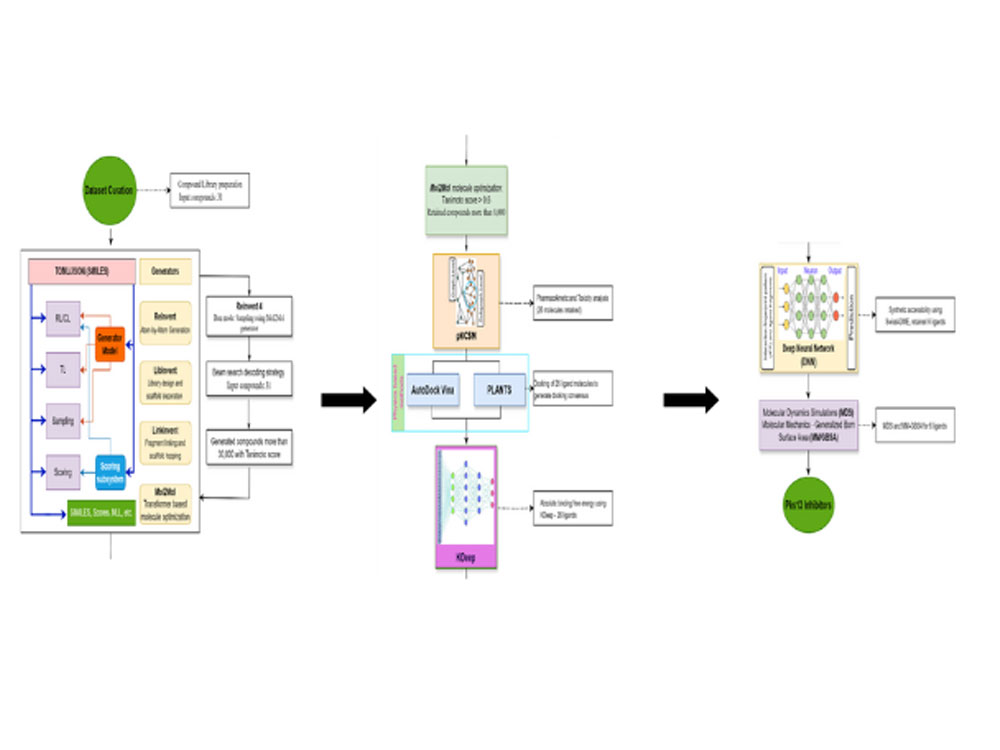
Identification of novel hit molecules targeting M. tuberculosis polyketide synthase 13 by combining generative AI and physics-based methods
Our latest research gives insight into the critical Pks13-TE domain of mycobacteria, employing a mix of AI and physics-based tools to identify new inhibitors. We utilized AI-ML tools such as Reinvent 4, pKCSM, KDeep, and SwissADME, alongside physics-based methods like AutoDock Vina, PLANTS, MDS, and MM-GBSA. Known Pks13-TE inhibitors were curated and used as input to generate novel inhibitors, which were then filtered through rigorous molecular docking and dynamics simulations. This meticulous process reduced the chemical space, retaining only the most promising compounds based on their interactions, stability, and binding energies. Further ADMET testing of these compounds revealed that ligands Mt1 to Mt6 possess excellent pharmacokinetic, pharmacodynamic, and toxicity profiles, making them strong candidates for drug development. The study “Identification of novel hit molecules targeting M. tuberculosis polyketide synthase 13 by combining generative AI and physics-based methods”, published in Computers in Biology and Medicine at Science Direct, showcases a robust framework for the discovery of potential Pks13-TE inhibitors.
Leave a Reply
Want to join the discussion?Feel free to contribute!